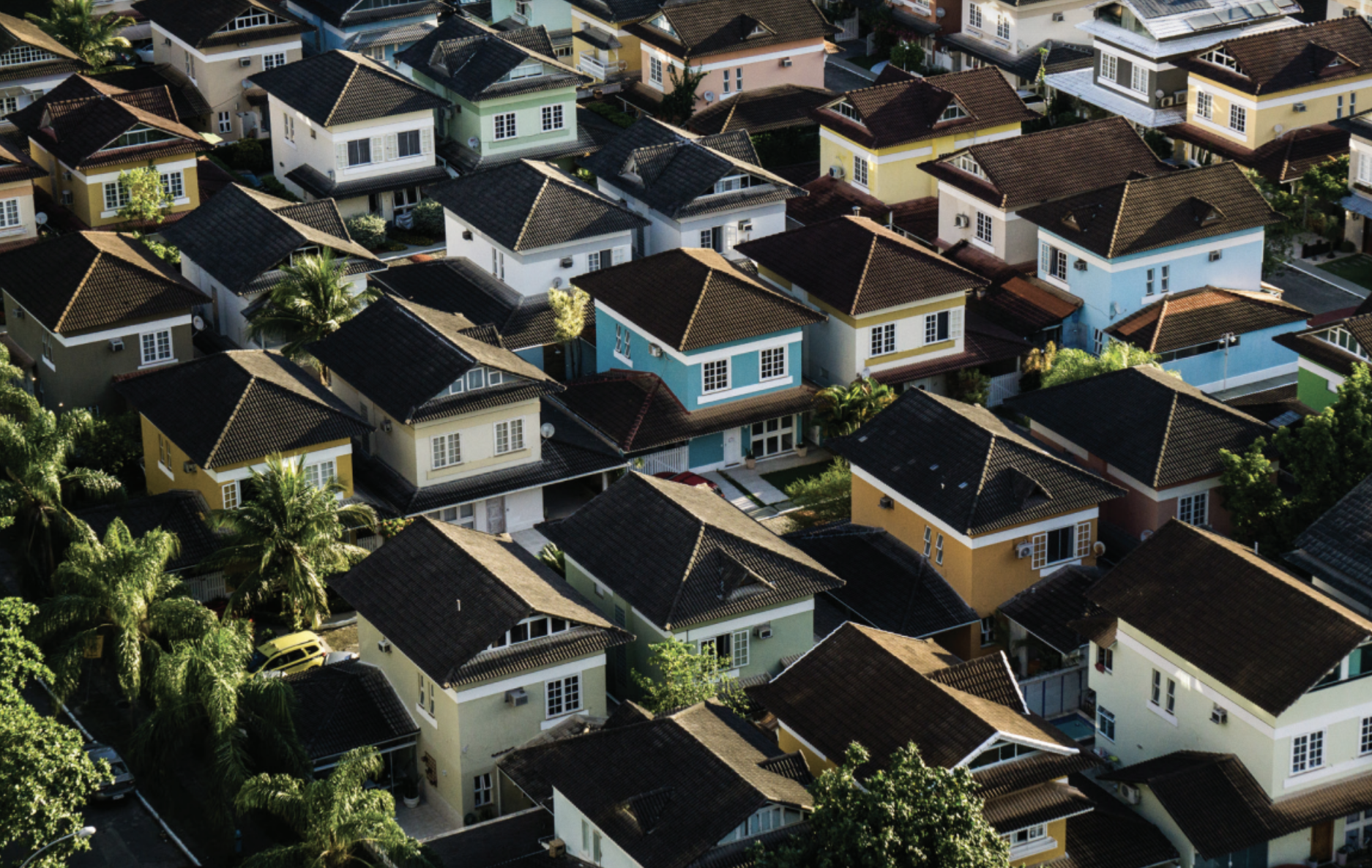
Fair Algorithmic Housing Loans
by Samara Trilling, with support from Madison Jacobs
Mortgage lenders increasingly use machine learning (ML) algorithms to make loan approval and pricing decisions. This has some positive effects: ML loan models can be up to 40 percent less discriminatory than face-to-face lending. Moreover, unlike human loan officers, algorithms can be tested for fairness before they’re released into the wild. But such decisions also present challenges: when ML models discriminate, they do so disproportionately against underbanked borrowers. In addition, it is often unclear how existing fair lending laws should be applied to algorithms, and they are updated too frequently for traditional fair lending audits to handle. To address these challenges, this project recommends that New York State banking regulators define a fairness metric for mortgage algorithms and pilot automated fair lending tests.
Click below to view various resources for understanding algorithmic loan fairness, including a policy brief, design principles for companies looking to make a policy change and educational materials to explain the issue.